In recent advancements in the field of artificial intelligence and natural language processing, one technique stands out for its ability to significantly enhance the capabilities of large language models (LLMs): , or RAG. RAG represents a novel approach where traditional generative models are augmented with retrieval capabilities, allowing them to access and utilize external knowledge sources to generate more accurate and contextually relevant responses.
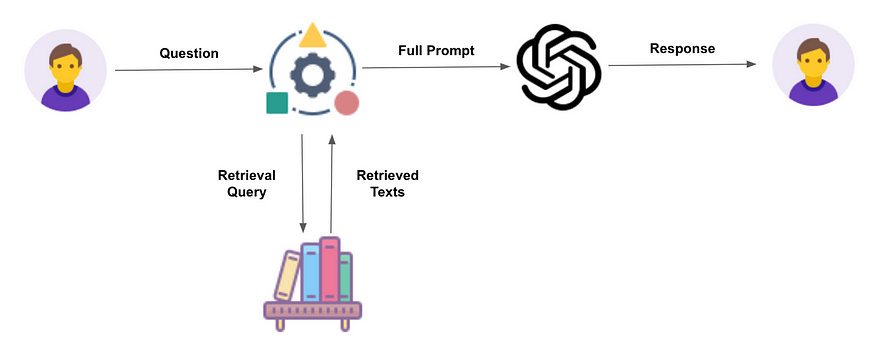
At its core, RAG operates through a systematic process designed to empower LLMs with the ability to reason and respond based on specific contexts or documents relevant to a query. Here’s how it works:
Understanding the Mechanics of RAG
- Retrieval of Relevant Information: When presented with a query, such as “Is there parking for employees?”, the RAG system first identifies potentially relevant documents or sources of information. For instance, in a corporate setting, documents related to facilities management might contain pertinent details about parking policies. The system sifts through these documents to pinpoint the most suitable ones that can provide an answer.
- Incorporation into the Prompt: The next step involves integrating the retrieved information into the prompt given to the LLM. Rather than relying solely on its pre-existing knowledge base or training data, the model is enriched with specific excerpts or data points extracted from the relevant documents. This augmented prompt provides the LLM with the necessary context to formulate a well-informed response.
- Generation of Response: Equipped with both the original query and the enriched prompt, the LLM leverages its generative capabilities to produce a nuanced and contextually aware answer. This response not only addresses the initial query but also draws directly from the retrieved information, ensuring a higher degree of accuracy and relevance.
- Enhanced User Experience: Beyond simply generating text, RAG applications often provide additional features such as linking back to the original source documents. This transparency allows users to verify the information provided and fosters trust in the accuracy of the AI-generated responses.
Practical Applications of RAG
The application of RAG extends across various domains and use cases:
- Enterprise Solutions: Companies are deploying RAG-powered systems to streamline internal operations, such as answering employee queries about policies or facilities.
- Educational Tools: Platforms like Coursera Coach utilize RAG to assist learners by answering questions based on course content, thereby enhancing the educational experience.
- Customer Support: Businesses integrate RAG into their customer service operations, enabling AI-powered chatbots to handle inquiries with greater efficiency and accuracy.
- Research and Development: In academic and research settings, RAG facilitates rapid access to relevant literature and data, aiding researchers in synthesizing information and generating insights.
Future Directions and Considerations
As RAG continues to evolve, there are ongoing efforts to refine its capabilities and address inherent challenges, such as ensuring the quality and reliability of retrieved information. The balance between generative prowess and retrieval accuracy remains a focal point for developers seeking to maximize the utility of AI-driven solutions.